Lowkey-Advanced Ridge Regression (Part II): Non-Zero Priors, Subset Shrinkage, Cluster Shrinkage
I Studied Regressions *Only* for 30 Days So You Don't Have To But You Have To Subscribe Part II
Code attached at the end of post.
Welcome back my Ridge-maximalist friends. Take pride in the fact that you are one of the fortunate ones that know the superiority of Ridge.
look at all the pathetic souls on a Sunday afternoon. mindlessly strolling around Regent’s Park like sheep taking pictures of flowers. they don’t have even the slightest idea that Ridge Regression dominates OLS for a range of values even when there is zero multicollinearity. pic.twitter.com/0sKuMRwFsM
— quantymacro (@quantymacro) April 7, 2024
Last time we covered lowkey-advanced stuffs. We now know the exact condition where Ridge will dominate OLS, we learned that Ridge favours true dense coefficients, among many other things.
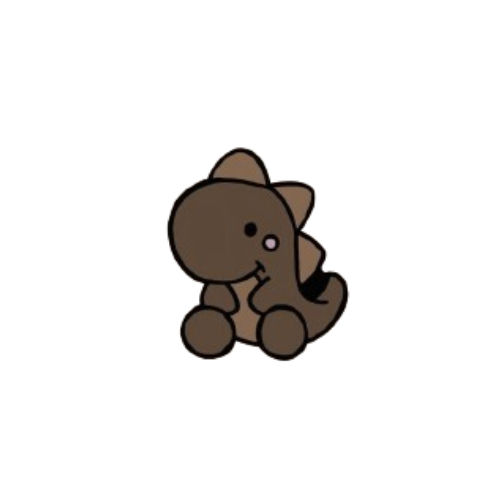
The article before was actually quite theoretical, but somehow was well received by the practitioners (which is something I appreciate a lot of course). In this article we will learn that Ridge Regression is a very versatile tool, and there are many knobs to turn to model the effects that we want.
Specifically, we will learn how:
Subset shrinkage in Ridge - featuring exclusive leaked DMs with @ryxcommar
Dealing with multiple clusters of features in Ridge
And bonus content:
How do you weight data points in your models? - featuring exclusive leaked DMs with @macrocephalopod